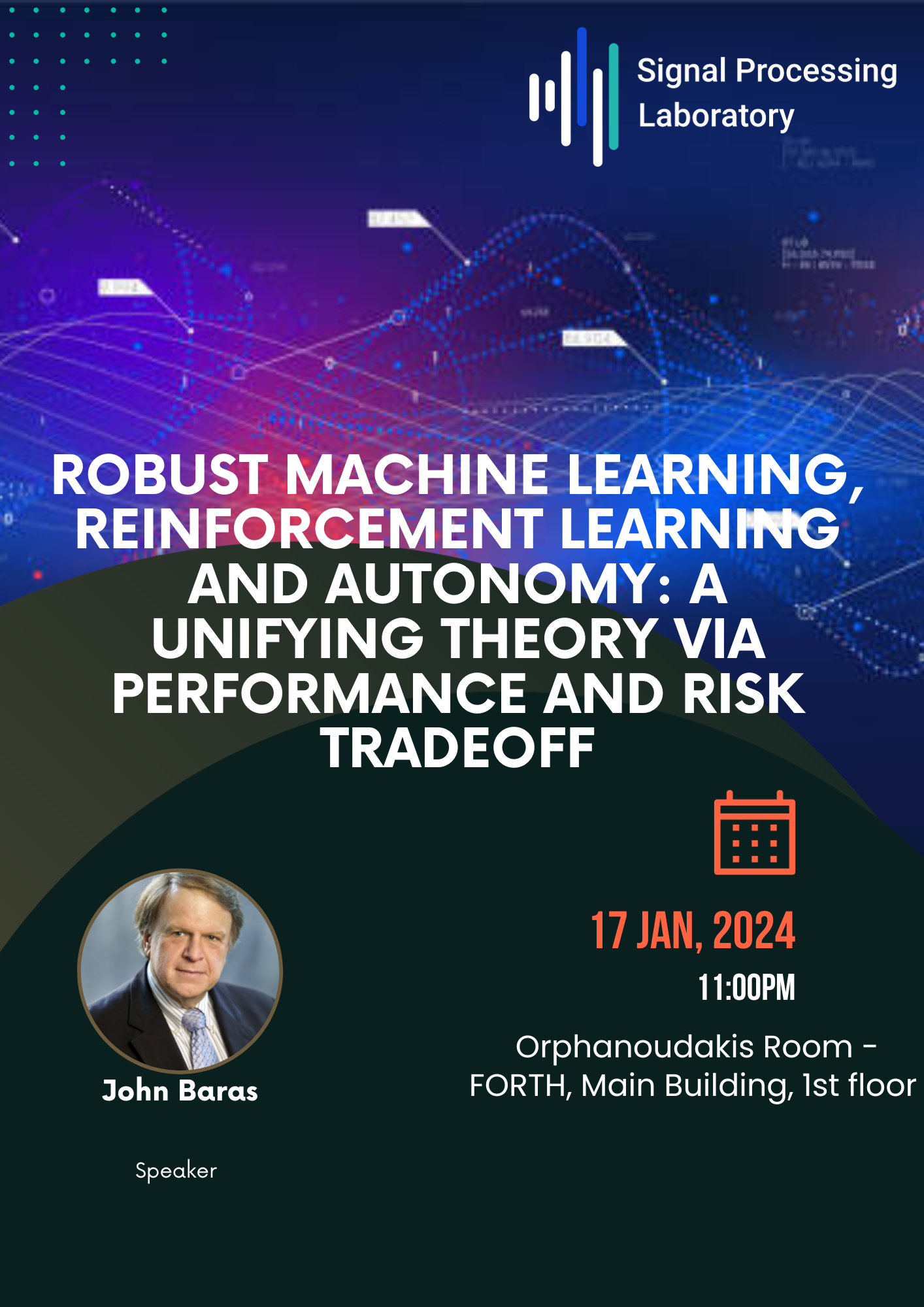
Speaker : John Baras
Date : 17.01.2024
Time: 11.00 to 12.30
Location : Orphanoudakis Room - FORTH, Main Building, 1st floor
Host : Prof. Panos Tsakalidis, SPL/ICS FORTH
Abstract:
Robustness is a fundamental concept in systems science and engineering. It is a critical consideration in all inference and decision-making problems. It has recently surfaced again in the context of machine learning (ML), reinforcement learning (RL) and artificial intelligence (AI). We describe a novel and unifying theory of robustness for ML/RL/AI emanating from our much earlier fundamental results on robust output feedback control for general systems (including nonlinear, HMM and set-valued). We briefly summarize this theory and the universal solution it provides consisting of two coupled HJB equations. These earlier results rigorously established the equivalence of three seemingly unrelated problems: the robust output feedback control problem, a partially observed differential game, and a partially observed risk sensitive stochastic control problem. We first show that the "four block" view of the above results leads naturally to a similar formulation of the robust ML problem, and to a rigorous path to analyze robustness and attack resiliency in ML. Then we describe a recent risk-sensitive approach, using an exponential criterion in deep learning, that explains the convergence of stochastic gradients despite over-parametrization. Finally, we describe our most recent results on robust and risk sensitive RL for control, using exponential rewards, that emerge from our earlier theory, with the important new extension that the models are now unknown. We show how all forms of regularized RL can be derived from our theory, including KL and Entropy regularization, relation to probabilistic graphical models, distributional robustness. The deeper reason for this unification emerges: it is the fundamental tradeoff between performance and risk measures in decision making, via rigorous duality. We close with open problems and future research directions.
Bio:
John S. Baras is a Distinguished University Professor, holding the Lockheed Martin Chair in Systems Engineering, in the Institute for Systems Research (ISR) and the ECE Department at the University of Maryland College Park (UMD). He received his Ph.D. degree in Applied Mathematics from Harvard University, in 1973, and he has been with UMD since then. From 1985 to 1991, he was the Founding Director of the ISR. Since 1992, he has been the Director of the Maryland Center for Hybrid Networks (HYNET), which he co-founded. He is a Fellow of IEEE (Life), SIAM, AAAS, NAI, IFAC, AMS, AIAA, Member of the National Academy of Inventors (NAI) and a Foreign Member of the Royal Swedish Academy of Engineering Sciences (IVA). Major honors and awards include the 1980 George Axelby Award from the IEEE Control Systems Society, the 2006 Leonard Abraham Prize from the IEEE Communications Society, the 2017 IEEE Simon Ramo Medal, the 2017 AACC Richard E. Bellman Control Heritage Award, and the 2018 AIAA Aerospace Communications Award. In 2016 he was inducted in the University of Maryland A. J. Clark School of Engineering Innovation Hall of Fame. In June 2018 he was awarded a Doctorate Honoris Causa by his alma mater the National Technical University of Athens, Greece. His research interests include systems, control, optimization, autonomy, machine learning, artificial intelligence, communication networks, applied mathematics, signal processing and understanding, robotics, computing architectures, formal methods, network security and trust, systems biology, healthcare management, model-based systems engineering. He has been awarded twenty patents and honored with many awards as innovator and leader of economic development.