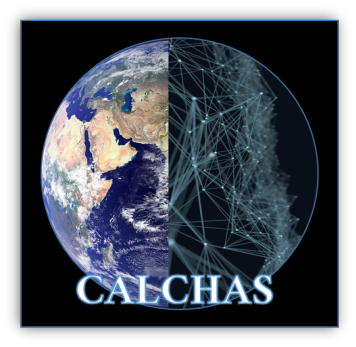
CALCHAS: Computational Intelligence for Multi-Source Remote Sensing Data Analytics
Dates: 11/2019 – 05/2022
Funded by: European Commission, H2020, Marie Sklodowska-Curie MSCA-IF-GF
Project Coordinator: P. Tsakalides and M. Moghaddam (USC)
Funding: € 215,492
Project Mission: Earth Observation (EO) is undergoing a radical transformation due to the massive volume of observations acquired by remote sensing and in-situ sensor networks. While satellites provide coarse-resolution, yet global-scale monitoring of environmental processes, in-situ sensor networks acquire high-accuracy localized measurements. Extracting information from spaceborne and ground-based instruments requires innovative solutions which will allow the autonomous integration of diverse in nature and scale observations in order to provide high-quality geophysical parameter estimation.
Visit Website
CALCHAS is a Marie Skłodowska-Curie Individual fellowship between:
the
Signal Processing Lab (SPL) of the Institute of Computer Science (ISC) at FORTH in Greece and
the MiXIL lab at the Electrical Engineering Department of the University of Southern California (USC) in Los Angeles, CA, USA.
Mission of the project
CALCHAS will demonstrate cutting edge technologies targeting three major factors towards the vision of fully automated multi-source EO data understanding, namely:
- the fusion of observations from different sources and modalities,
- the efficient aggregation of the sampling scales associated with spaceborne and in-situ measurements,
- the generation of novel value-added satellite-derived products
To that end, the paradigm shifting signal processing and learning framework of Deep Learning will be utilized and extended through powerful mathematical tools and appropriate methodologies like supervised and generative learning, dramatically extending the current scope of single-source data analysis. The developed framework will be employed for analyzing time-series of measurements from active and passive microwave and multispectral spaceborne imaging instruments (SMAP, SMOS, and Sentinels), and in-situ sensor measurements, targeting the high-accuracy spatial and temporal resolution enhancement for observations and soil moisture estimation.