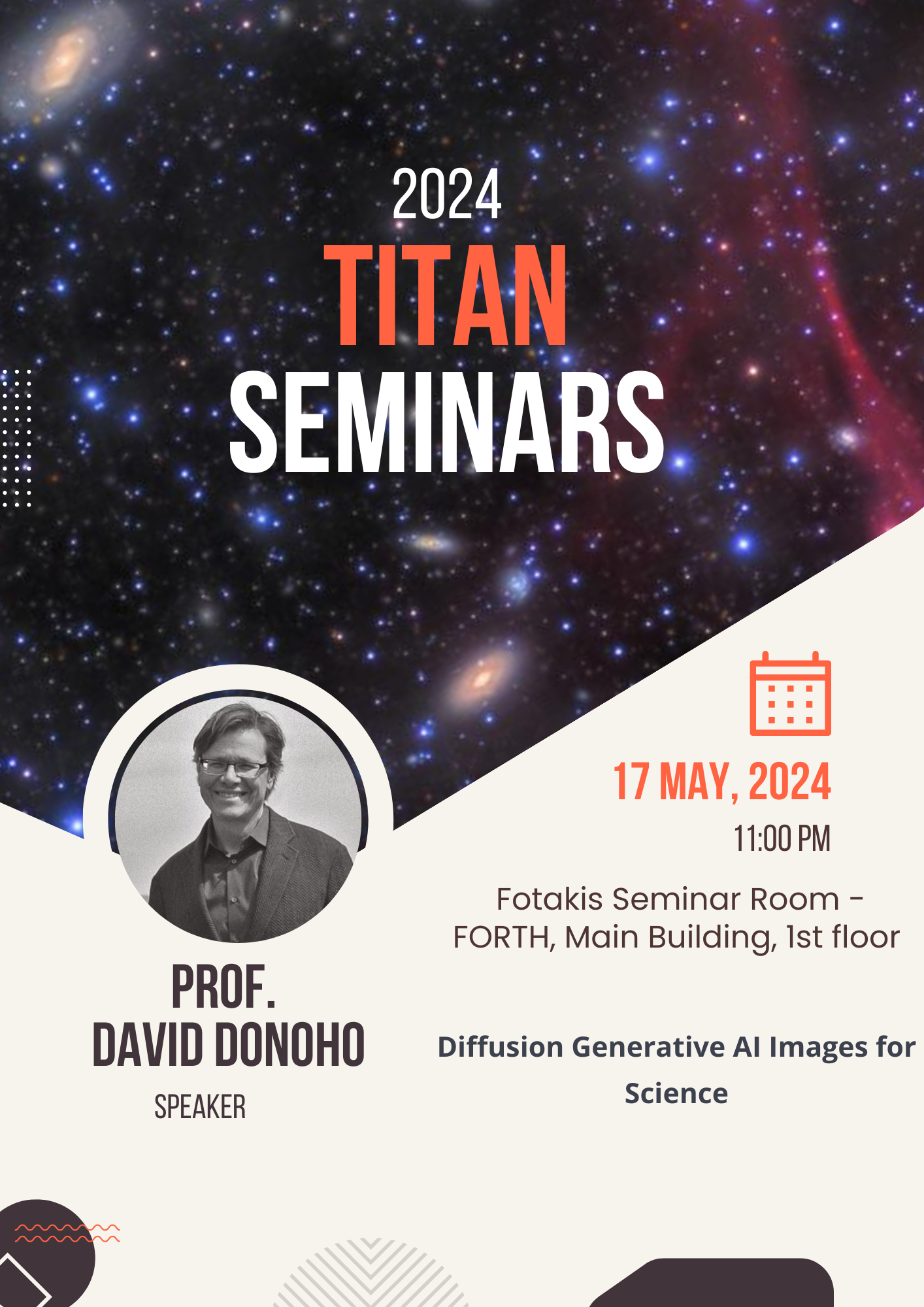
Speaker : David Donoho, Professor of Statistics, Stanford University
Date : 2024-05-17
Time: 11:00 - 12:30
Location : Fotakis Seminar Room - FORTH, Main Building, 1st floor
Host : Jean-Luc Starck and Panagiotis Tsakalides
Abstract:
Recently Generative AI has completely transformed the landscape of “consumer level” artwork. For example, one can make quite attractive posters to inform people of lectures and meetings, or to be tweeted out – simply providing some informal text as a prompt. It is hard to imagine a commercial artist doing something so vivid so quickly starting from the same short text fragment. With all this excitement, it is easy to imagine that generative AI can benefit science .... but will it really?
We will review instances of Generative AI based on the so-called Stable Diffusion algorithm and its relatives and variations. This class of algorithms is based on iterative applications of denoising and has the “wow” effect that an interesting image emerges from noise. We will review the basic workflow and the developing landscape of theoretical explanations of why this workflow can be successful. We will then survey instances where generative AI is starting to be used in scientific image-related processing, and discuss the critical issues still facing this technology.
This is joint work with Apratim Dey, XY Han, and Joshua Kazdan of Stanford and Vardan Papyan of University of Toronto.
Bio:
David Donoho has studied the exploitation of sparse signals in signal recovery, including for denoising, superresolution, and solution of underdetermined equations. His research with collaborators showed that ell-1 penalization was an effective and even optimal way to exploit sparsity of the object to be recovered. He coined the notion of compressed sensing which has impacted many scientific and technical fields, including magnetic resonance imaging in medicine, where it has been implemented in FDA-approved medical imaging protocols and is already used in millions of actual patient MRIs.
In recent years David and his postdocs and students have been studying large-scale covariance matrix estimation, large-scale matrix denoising, detection of rare and weak signals among many pure noise non-signals, compressed sensing and related scientific imaging problems, and most recently, empirical deep learning.